Application of artificial intelligence in drug development
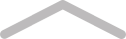
Application of artificial intelligence in drug development
Through machine learning, evolutionary computing, image recognition, natural language processing and cognitive computing, artificial intelligence (AI) can be applied in the following fields: Drug Targets Development, Drug Mining, Compound Screening, Predication of ADMET Properties, Crystal Structure Prediction, Pathophysiology, and Drug Repurposing.
The key to research and development of new drugs is to find, determine and prepare drug targets. Drug targets refer to the binding sites of drugs in the body, including biological macromolecules, such as loci, receptors, enzymes, ion channels and nucleic acids. Identifying new and effective drug targets is the top priority of new drug development. Therefore, the work involved in discovering and verifying a large number of molecular targets has greatly increased the burden of drug development. Machine learning algorithms can be fully applied to the old single-target drug discovery project. Researchers first studied the possibility of selective binding of targets to balanced small molecules to determine which targets are easiest to handle chemically. For bispecific small molecules, the design process is similar to a single target drug. The key difference is that efficacy must meet two different goals at the same time.
Drug mining
AI can generate useful information from a large number of scientific papers, patents, clinical trial information, and unstructured information. Through deep learning optimization of natural language processing algorithms, it analyzes and understands contextual information, and then further learns, explores, creates and translates the knowledge it has learned to produce unique conclusions. This technology makes possible scientific discoveries that were previously impossible by searching for possible missing connections. It can automatically extract pharmacy and medical knowledge, find out related relationships and propose corresponding candidate drugs, and further screen for molecular structures that are effective for certain diseases, enabling scientists to develop new drugs more effectively. In 2016, Benevolent AI used artificial intelligence algorithms to identify 5 drugs within one week for the treatment of amyotrophic lateral sclerosis. Benevolent AI uses AI algorithm modeling to confirm the compound's potential effect on sleep, which is a great opportunity to solve the symptoms of Parkinson's disease-related sleepiness. The company's current drug development product portfolio shows that it can shorten the time for early drug development by 4 years and may increase the average efficiency of drug development by 60% throughout the drug development process.
Compound screening
Compound screening refers to the method of selecting compounds with higher activity on specific targets from a large number of compounds through standardized experimental methods. To choose molecules that match the activity index from tens of thousands of compounds usually requires a long time and high cos. AI systems have been developed based on convolutional neural networks, which has learned a lot of chemistry knowledge and research data. The system analyzes the structure-activity relationship of compounds, determines the basic modules in medicinal chemistry, and is used for the discovery and risk assessment of new drugs.
The properties of ADMET are the most important reference index to measure the drug-making properties of compounds. ADMET prediction is a very important method in contemporary drug design and drug screening. The early ADMET characteristics of drugs mainly used human or humanized tissue functional proteins as drug targets, and in vitro research technology combined with computer simulation to study the interaction between drugs and in vivo biophysical and biochemical barrier factors. In order to further improve the accuracy of ADMET properties prediction, some companies have explored the effective extraction of structural features (including the processing of small molecules and protein structures) through deep neural network algorithms, speeding up the early detection and screening process of drugs.
Prediction of Drug Crystal Form
Polymorphism is a phenomenon in which a substance can exist in two or more different crystal structures. For chemical drugs, almost all solid drugs have polymorphism. Since the change of crystal form can change many physical and chemical properties of solid chemical drugs, there are several drugs that have failed to market due to crystal form problems. Therefore, crystal form prediction is of great significance in the pharmaceutical industry. Using artificial intelligence to dynamically configure drug crystal forms can completely predict all possible crystal forms of small molecule drugs. Compared with traditional drug crystal form development, pharmaceutical companies do not have to worry about the lack of important crystal forms. In addition, crystal form prediction technology greatly shortens the development cycle of crystals and reduces costs.
Over the years, researchers have gradually realized that the best strategy to improve drug discovery efficacy is to treat certain diseases based on existing drugs, discover new indications and use them to treat another disease. In cooperation with IBM Watson for Drug Discovery, Dr. Visanji used Watson's powerful literature reading and cognitive reasoning skills to screen out 3,500 drugs within a few minutes and arrange them in the order of best match. The researchers then proposed 6 candidate drugs based on this "drug ranking table" and tested them in the laboratory. The first drug (which has been approved by the FDA, but the indication does not include Parkinson's disease) has been initially verified in animal experiments.
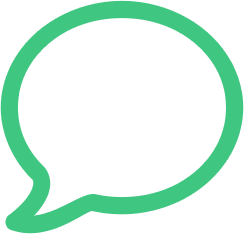